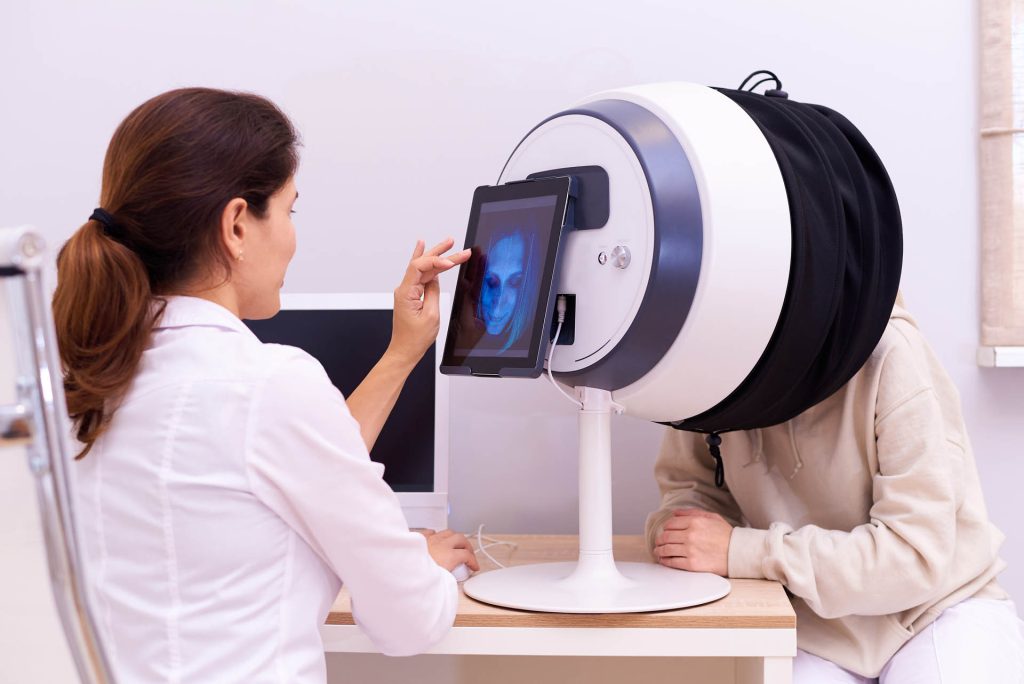
We are transforming the landscape of medical imaging through artificial intelligence and machine learning. Our research focuses on developing advanced imaging solutions that enhance diagnostic accuracy, improve patient outcomes, and make healthcare more accessible worldwide.
Ongoing Research
- Automated Disease Detection and Diagnosis
- Objective: Build AI models capable of detecting diseases such as cancer, cardiovascular conditions, and neurological disorders with high precision.
- Progress: Developed deep learning algorithms that achieve 95% accuracy in tumor detection across multiple imaging modalities, including MRI, CT, and ultrasound.
- Image Segmentation and Annotation
- Objective: Automate the segmentation of organs, tissues, and anomalies to assist radiologists.
- Progress: Created a U-Net-based model that reduces segmentation time by 70%, providing pixel-perfect annotations for clinical applications.
- Multi-Modal Imaging Analysis
- Objective: Integrate data from diverse imaging modalities (e.g., PET, CT, and MRI) for a comprehensive diagnostic view.
- Progress: Implemented a multi-modal fusion network that combines imaging data, achieving 30% better diagnostic accuracy than single-modality analysis.
- Low-Cost Imaging and Enhancement
- Objective: Develop AI-driven imaging systems for low-resource settings, focusing on enhancing low-resolution and noisy scans.
- Progress: Successfully deployed AI-powered denoising techniques that improve the quality of portable X-rays, reducing the need for repeat imaging.
- Predictive Analytics and Risk Assessment
- Objective: Use medical imaging data to predict disease progression and treatment outcomes.
- Progress: Designed a predictive AI model for stroke risk assessment that integrates imaging biomarkers, demonstrating an 85% prediction accuracy.
Key Results and Milestones
- Cancer Detection: Our AI models have been validated in clinical settings, identifying breast and lung cancer at early stages with a sensitivity exceeding 92%.
- Enhanced Diagnostic Efficiency: Reduced radiologist workload by integrating automated image analysis tools, allowing faster report generation.
- Global Impact: Collaborated with NGOs to deploy low-cost AI imaging tools in underserved regions, providing affordable diagnostic support to remote communities.
- Regulatory Milestones: Achieved FDA clearance for our AI-powered diagnostic tool for retinal disease screening.
Future Directions
As we advance, our goals include:
- Improving interpretability and explainability of AI models for medical imaging.
- Expanding our research to include rare diseases and specialized imaging techniques.
- Enhancing collaboration with healthcare providers to accelerate clinical translation.
- Fostering ethical AI practices to ensure unbiased and equitable diagnostic systems.